Developers’ voice Takashi Togami
Not a technical point of view, think what you have to do from the plant perspectives
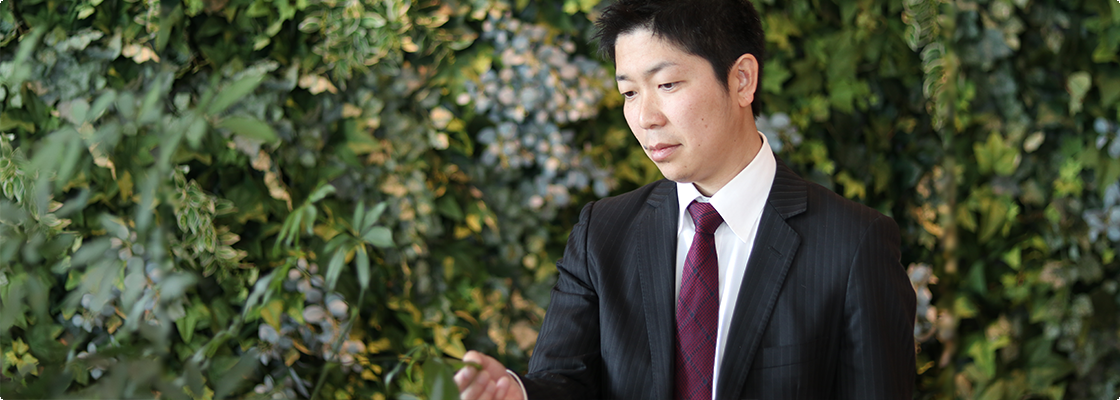
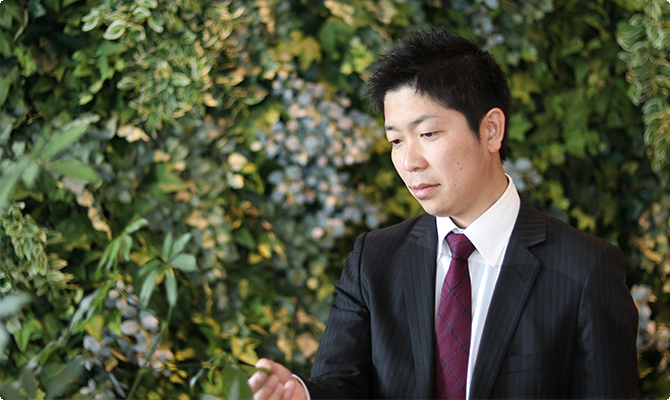
e-kakashi has been accepted in the field of agriculture because it was developed with a focus on plant perspectives
The most important point in the development of e-kakashi was the “plant perspective”. In the first place, e-kakashi is a mass of technologies such as sensors, ICTs, and else. I think the reason why such technologies have not been used in the field of agriculture so far is because there was no “plant perspective”. For example, water is generally important for growing plants.
I say water is important, but what is important about water? The amount? Or the quality? If the amount is important, how much is the optimal amount? That’s the point you need to think deeply. The reason why plants need water is for photosynthesis, and it is also necessary for sucking and carrying nutrients from the roots.
It is also necessary to maintain body temperature. Water is important for plants, but you must think about why it is important. This is one of the development philosophies of e-kakashi that we always think from a plant perspective. In addition to the plant's perspective, we have to consider market needs, that is the quality of crops. There are various demands such as wanting to grow crops larger, sweetening, or harvesting them quickly. Until now, farmers had to rely on experience and intuition to grow crops. However, in the academic world, there is already certain knowledge such as plant physiology and plant pathology.
We will combine scientific evidence with experience and intuition using environmental data and provide them in a form that is easier to use for end users. That’s why I think e-kakashi would be useful.
Absorb data other than cultivation data, and grow e-kakashi something that anyone can’t imagine with current status
Now, some farmers say “e-kakashi is maniac”. They think e-kakashi is amazing, but they feel that much data is not needed. But personally, I still think it’s not enough.
For example, in terms of “temperature”, accumulated temperature is a very important index for growing crops. However, there is air temperature and soil temperature. Also, paddy fields also have water temperature. The air temperature meaning differs depending on whether the temperature is close to the ground or 50 cm above the ground. The soil temperature meaning differs whether the temperature is soil surface or 15 cm underground. All of that will be converted into data.
The data is analyzed in an easy-to-understand manner and visualized. The important thing is not "I can do this now, let's do it", but "I have to do this, so let's do it".
What I want to work on is not only the refinement of the data that I have just taken, but also the records of human work performed there. Cultivation data, crop data, and human work records are separate and have not been integrated so far. Furthermore, I want to collect and analyze soil data and reflect it in the analysis results. I think that it is meaningless unless information on microorganisms contained in the soil is converted into data. It's hard to achieve, but I think it's essential if I think about the future of agriculture. As a result, in 5 or 10 years, e-kakashi would be the great solution that anyone cannot imagine now.
PROFILE
- Takashi Togami Ph.D.
Greein Inc.
Representative Director and CEO - Dr. Togami graduated with a B.S. in applied sciences from Charles Sturt University in New South Wales, Australia, followed by a master’s degree from Mie University in Japan, where he researched agricultural information and communication technology. He obtained a doctorate in bioresources science in 2012, also from Mie University, researching sensor networks and information utilization on farms. In December 2012, Dr. Togami received an honorable mention at theInternational Commission of Agricultural and Biosystems Engineering subcommittee of the Science Council of Japan for his work on a wireless sensor network for precise soil water management in an orchard. He joined SoftBank Mobile(now:SoftBank Crop.) in 2013, where he currently leads technology development projects related to agricultural Internet-of-Things technology and expertise.
Developers’ voice Kyosuke Yamamoto
e-kakashi's hybrid AI applies plant physiology, and enables meaningful predictions with limited volume of data
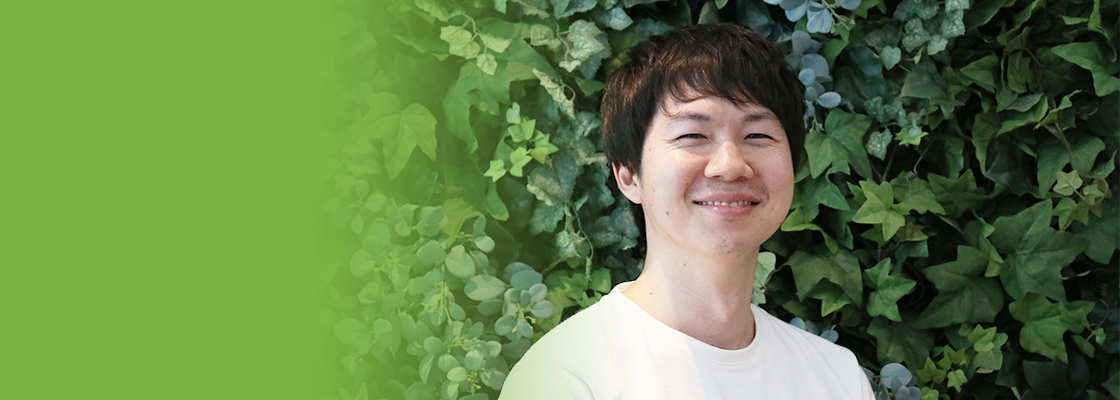
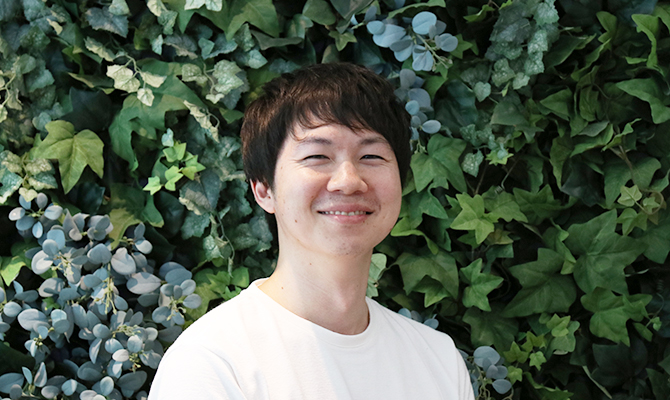
Adding the essence of plant physiology to the technology of information science
In recent years, AI and deep learning have become buzzwords. Such technologies have been used in a variety of applications in the agricultural world. However, personally, I think there is a significant hurdle in applying the technology developed in information science to agriculture as it is. There are various reasons for that, but the most sgnificant point is the difficulty of data collection in agriculture. In particular, in the case of plant growth data, it could take more than a year to collect just one record. On the other hand, for example, millions of data are already prepared for the purpose of image analysis, and such amount of data is definitely required to achieve the greate performance of deep learning. I think that one of the challenges of AI utilization in agriculture is how to obtain new knowledge from machine learnings with small data.
e-kakashi develops analytical technology that can produce meaningful predictions even with the small data by incorporating the essence of plant physiology (=known facts), into the technology of information science such as AI. Using this analysis technology, we can propose an environmental control method to increase yield and profit, from the cultivation environment data and growth data in a glasshouse. As explained earlier, our technology does not require a large amount of historical data, decades of data for instance, but you can realize the benefits of our technology from the first year. In addition, our analysis technology is evolving day by day while incorporating large amounts of sensor data and newly found knowledges about plant physiology.
Agricultural information platform
We are not the serviser of hardware or software. We are developing a platform that connects farmers in various layers. As a first step, we built the “cultivation technology platform”. There exist many communications about cultivation skills and knowledges between farmers in various forms. Our platform aggregates them in one place, and provides various tools, data and analysis results to facilitate the information transfer.
Of course, e-kakashi provides not just a platform for cultivation technology. We will develop “Agricultural information platform” that facilitates various transactions related to agriculture, such as farmer's operation history, plant growth information, material buying and selling, product distribution and sales etc. Our platform provides new values generated from the interactions to every users.
PROFILE
- Kyosuke Yamamoto Ph.D. Greein Inc. Director CTO
- Engaged in research on high-speed phenotyping of plants using image analysis and machine learning at the Graduate School of Mie University and the University of Tokyo. In 2015, he received a doctorate in agriculture from the University of Tokyo. From the same year, he was in charge of R&D of e-kakashi.
- Published papers
- Yamamoto, K. Distillation of crop models to learn plant physiology theories using machine learning. PLoS One 14, e0217075 (2019).
- Yamamoto, K., Togami, T. & Yamaguchi, N. Super-Resolution of Plant Disease Images for the Acceleration of Image-based Phenotyping and Vigor Diagnosis in Agriculture. Sensors 17, 2557 (2017).
- Yamamoto, K., Togami, T., Yamaguchi, N. & Ninomiya, S. Machine Learning-Based Calibration of Low-Cost Air Temperature Sensors Using Environmental Data. Sensors 17, 1290 (2017).
- Yamamoto, K., Guo, W. & Ninomiya, S. Node Detection and Internode Length Estimation of Tomato Seedlings Based on Image Analysis and Machine Learning. Sensors 16, 1044 (2016).
- Yamamoto, K. et al. Strawberry cultivar identification and quality evaluation on the basis of multiple fruit appearance features. Comput. Electron. Agric. 110, 233–240 (2015).
- Yamamoto, K., Guo, W., Yoshioka, Y. & Ninomiya, S. On Plant Detection of Intact Tomato Fruits Using Image Analysis and Machine Learning Methods. Sensors 14, 12191–12206 (2014).